
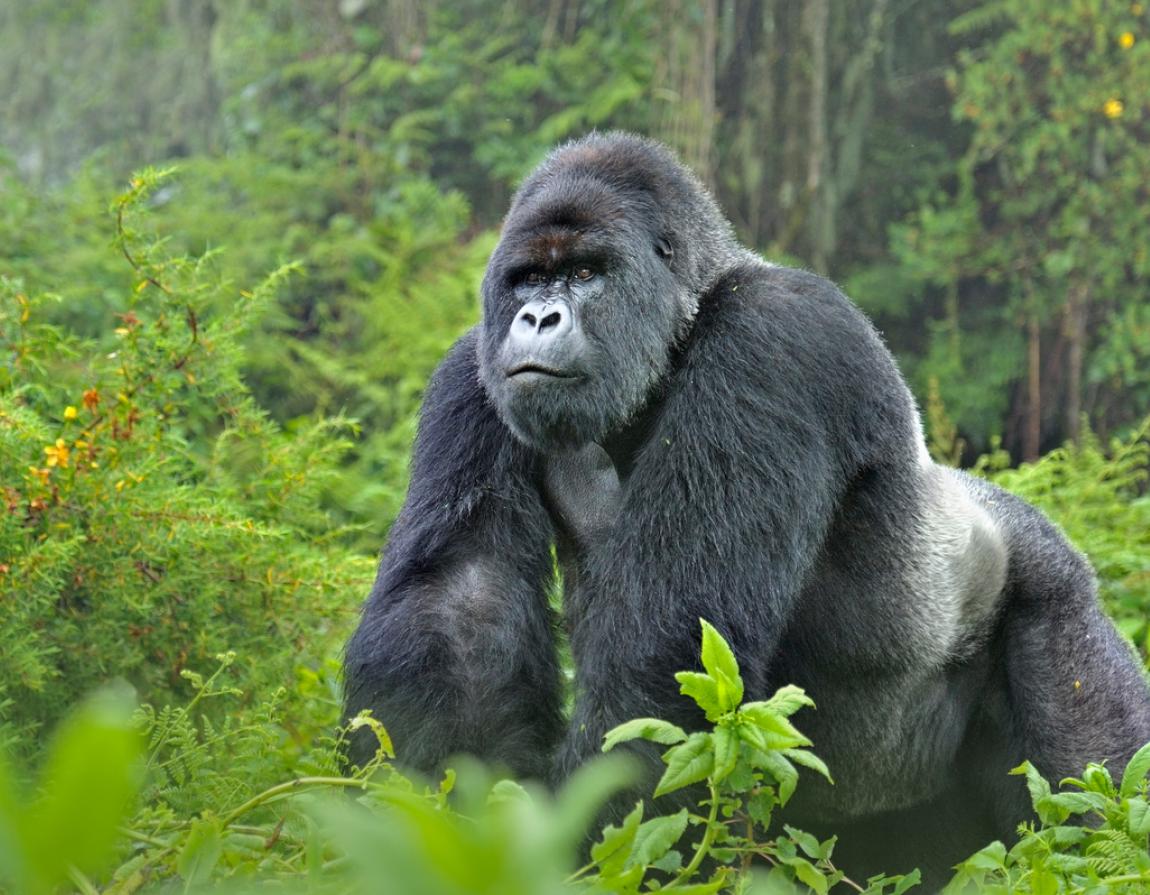
Google took a similar approach with its voice-search feature that lets users ask questions verbally rather than by typing search queries. Google said that as more images are loaded into Google Photos and more people correct mistaken tags, its algorithms will get better at categorizing photos. “The bias of the Internet reflects the bias of society,” she said.

Some systems struggle to recognize non-white people because they were trained on Internet images which are overwhelmingly white, she explained. Now, most systems are set up to make their best guess at a label, even if they’re not 100% sure, Hodjat explained.Īrtificial intelligence expert Vivienne Ming said machine-learning systems often reflect biases in the real world. But such systems also have to be trained to be more cautious in certain settings. They can’t zoom in and understand this type of context.”įeeding more pictures of gorillas into Google’s machine learning system would help. “Humans are very sensitive and zoom in on certain differences that are important to us culturally,” Hodjat said. Google’s system may not have “seen” enough pictures of gorillas to learn the differences – and wouldn’t understand the significance of such a mistake, he said. He said machine-learning systems don’t understand the difference between mistaking a chimp for a gorilla, which may be OK, and mislabeling a human as a gorilla, which is offensive. “We need to fundamentally change machine learning systems to feed in more context so they can understand cultural sensitivities that are important to humans,” said Babak Hodjat, chief scientist at Sentient Technologies, an artificial-intelligence startup. The algorithms for photo recognition need to be more accurate. Rather, it is taught to recognize generally that there’s an object and decide what to do next based on the object’s motion and speed. The machine-learning system in Google’s cars isn’t taught to recognize specific objects. Google’s self-driving cars, which are being tested on public roads, use the technology to recognize objects and decide whether to stop, avoid or continue. Getting this technology right is increasingly important as machine learning is used for more everyday tasks. But the “gorillas” tag shines a harsh light on the system’s shortcomings. When it launched the Photos app, Google acknowledged that it was imperfect. A Google spokeswoman said at the time that it was “nearly impossible to have 100% accuracy.” But the system missed some inappropriate content, sparking complaints.
#GORILLA IMAGES MANUAL#
Google launched a YouTube Kids app earlier this year that aims to exclude adult content using a combination of automatic filters, user feedback and manual reviews. This gets products out to users fast, but risks upsetting consumers if bugs are major.
#GORILLA IMAGES UPDATE#
Google likes to release software that may still have flaws and then update to fix any problems. The episode shows the shortcomings of artificial intelligence and machine learning, especially when used for consumers.
#GORILLA IMAGES SKIN#
Google is working to improve its recognition of skin tones and will be more careful about its labels for people in photos, he added. But we’re very much on it,” Yonatan Zunger, chief architect of social at Google, wrote on Twitter in reply to Alciné. “Lots of work being done, and lots still to be done. The company has removed the gorilla categories, so those suggestions will no longer appear. When users start a search, Google suggests categories developed from machine learning, the science of training computers to perform human tasks such as labeling. The gorilla tags turned up in the search feature of the Google Photos app, which the company released a few weeks ago. “There is still clearly a lot of work to do with automatic image labeling, and we’re looking at how we can prevent these types of mistakes from happening in the future.” “We’re appalled and genuinely sorry that this happened,” a company spokeswoman said.
